AI-Powered Tools for User Experience Research
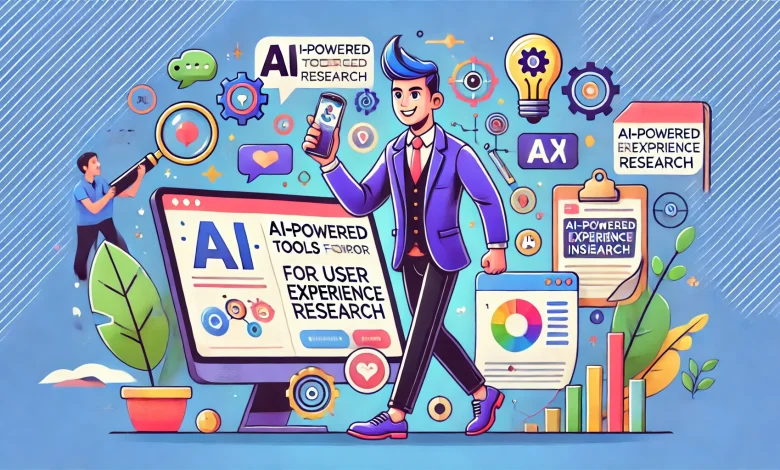
In the fast-evolving world of product design, artificial intelligence (AI) has become an indispensable tool for user experience (UX) research.
AI-powered tools not only accelerate data collection and analysis but also offer deeper insights into user behavior, enabling product managers and UX designers to make data-driven decisions that drive user satisfaction and business growth.
This article explores how AI-powered tools are transforming UX research. From foundational concepts to advanced techniques, we’ll dive into how AI helps teams optimize user experiences, align with business objectives, and stay ahead of the competition.
Introduction to AI in User Experience (UX) Research
AI in UX research leverages technologies like machine learning, natural language processing (NLP), and computer vision to automate data collection, analyze user behavior, and generate actionable insights. This approach helps UX teams understand how users interact with products in real time, enabling faster, more informed design decisions.
By reducing manual research efforts, AI allows teams to focus on strategic design improvements that directly impact user satisfaction and product success.
Why AI is Essential for Modern UX Research
The importance of AI in modern UX research lies in its ability to:
- Accelerate the research process: AI-powered tools can analyze massive datasets in seconds, providing real-time insights.
- Reduce human bias: AI delivers objective, data-driven conclusions, minimizing the subjectivity often present in traditional research methods.
- Uncover deeper insights: By processing vast amounts of behavioral data, AI reveals patterns and preferences that inform more user-centric design decisions.
Business Impact: AI-driven research not only enhances UX but also leads to improved business outcomes, such as faster go-to-market strategies, higher user retention, and increased product engagement.
Understanding the Basics of AI-Powered UX Tools
AI technologies like machine learning, NLP, and computer vision are foundational to UX research:
- Machine learning: Analyzes user interactions to identify patterns and predict future behaviors, helping teams design more intuitive experiences.
- NLP: Processes and categorizes qualitative feedback, such as surveys and reviews, identifying common themes and user concerns.
- Computer vision: Analyzes visual data, such as where users focus their attention on a screen, offering insights into optimizing visual elements.
Strategic Insight: These AI technologies allow UX teams to create more personalized, responsive designs that adapt to user needs, improving overall product performance.
Benefits of Using AI in UX Research
AI-powered tools offer several key advantages for UX teams:
- Real-time insights: AI provides instant feedback on user interactions, allowing for faster iteration.
- Improved user segmentation: AI can segment users based on behavior, preferences, and demographics, offering targeted insights.
- Handling complex datasets: AI processes large volumes of data efficiently, making it easier to manage and interpret user behavior across diverse platforms.
Key AI-Powered UX Research Tools in the Market
Several AI-powered tools are revolutionizing UX research:
- UserTesting: Captures user behavior with AI-assisted analysis for real-time insights.
- Hotjar: Uses heatmaps and behavior tracking to visualize how users interact with web pages.
- Lookback: Provides remote user testing with AI-driven analytics to identify friction points.
- Optimal Workshop: Combines card sorting and tree testing with AI analysis to improve information architecture.
These tools help UX teams streamline their research processes and make data-driven design decisions.
AI for Behavioral Analytics in UX Research
AI-powered behavioral analytics tracks and analyzes how users navigate products. It identifies common patterns, friction points, and drop-offs in user journeys:
- Pain point identification: AI highlights where users struggle most, guiding UX teams to focus on high-impact design improvements.
- Optimizing user flows: By tracking user behaviors, AI reveals the most efficient navigation paths, enabling more intuitive interfaces.
Business Impact: Improving user flows and resolving pain points leads to higher engagement, increased conversions, and reduced churn.
Natural Language Processing (NLP) for Analyzing User Feedback
NLP allows AI to categorize and analyze qualitative feedback from users, such as survey responses or customer reviews. It helps:
- Identify recurring themes in user feedback, such as dissatisfaction with a particular feature.
- Gauge user sentiment by analyzing the tone and emotion in feedback, providing deeper insights into user satisfaction.
Strategic Insight: NLP enables faster and more accurate processing of qualitative data, allowing UX teams to address user concerns proactively.
AI-Powered Heatmaps for Visualizing User Interaction
AI-driven heatmaps provide a visual representation of where users engage most with a product. They help UX teams:
- Identify high-engagement areas: AI pinpoints which elements users interact with most, helping to optimize those areas for better usability.
- Improve design layouts: Heatmaps show which elements are being overlooked, guiding design adjustments to increase visibility and engagement.
Example: An e-commerce site uses AI-powered heatmaps to optimize product placements, resulting in a 20% increase in conversions by making popular items more visible.
Predictive Analytics in UX Research
AI-powered predictive analytics anticipates user behavior, allowing UX teams to design more intuitive experiences:
- Predicting user drop-offs: AI analyzes user behavior to predict when users are likely to abandon a task, enabling preemptive design improvements.
- Proactive design: By anticipating user needs, AI helps UX teams create interfaces that reduce cognitive load and improve satisfaction.
Business Impact: Predictive analytics reduces user friction and increases engagement, leading to higher customer retention rates.
AI for Multivariate Testing in UX Research
Multivariate testing with AI allows teams to test multiple design variations simultaneously, optimizing layouts, features, and functionality based on user behavior data:
- Simultaneous testing of variables: AI-driven tools test different combinations of design elements, accelerating the optimization process.
- Automated results: AI identifies the best-performing designs based on user interactions, reducing the time needed to achieve optimal user experiences.
Machine Learning for Deep User Insights
Machine learning algorithms analyze extensive datasets to uncover deep insights into user behavior:
- Behavioral trends: Machine learning tracks long-term trends, allowing teams to stay ahead of emerging user preferences and needs.
- Long-term UX strategy: By understanding user behavior over time, UX teams can create forward-looking strategies that enhance product stickiness and retention.
AI for Sentiment Analysis in UX Research
AI-driven sentiment analysis evaluates user feedback to determine the emotional tone of reviews, social media mentions, and surveys:
- Measuring satisfaction: Sentiment analysis quantifies user satisfaction and highlights areas for improvement.
- Data-driven prioritization: Teams can prioritize UX changes based on sentiment, ensuring the most pressing user concerns are addressed first.
Strategic Insight: Sentiment analysis helps align product design with user emotions, driving positive brand experiences.
Ethical Considerations for AI in UX Research
When using AI in UX research, ethical considerations must be addressed:
- Data privacy: Ensure that user data is handled responsibly and in compliance with regulations such as GDPR.
- Bias mitigation: AI models must be regularly tested for biases to ensure they produce fair, unbiased insights.
- Transparency: Product managers should be transparent about how AI-driven insights are used, maintaining user trust.
Business Impact: Addressing ethical considerations builds trust with users and ensures compliance with legal standards, mitigating reputational risks.
Best Practices for Implementing AI-Powered UX Research Tools
To integrate AI-powered tools into UX workflows:
- Start small: Begin with pilot projects to experiment with AI-driven insights.
- Ensure cross-functional collaboration: Collaborate closely with data scientists, UX researchers, and product managers to fully leverage AI capabilities.
- Iterate frequently: Use AI to gather real-time insights, enabling faster iteration of designs and improvements.
Case Study: How AI Improved UX Research for a Major Tech Company
A major tech company integrated AI-powered tools into its UX research process, resulting in:
- Faster data collection: AI-driven user behavior analysis reduced research timelines by 50%.
- Improved user satisfaction: Predictive analytics helped the company anticipate user needs, resulting in a 20% improvement in user satisfaction scores.
AI for Identifying and Reducing UX Pain Points
AI can automatically detect pain points in the user experience:
- Identifying drop-off rates: AI analyzes patterns in user data to find areas where users struggle.
- Actionable recommendations: AI provides suggestions for addressing pain points, leading to smoother user interactions.
Creating a Continuous Feedback Loop with AI in UX Research
AI-powered tools enable continuous feedback loops:
- Real-time data collection: AI gathers ongoing user feedback, providing insights as users interact with the product.
- Continuous improvement: This real-time feedback allows for immediate design improvements, creating a more responsive and user-friendly product.
Future Trends in AI-Powered UX Research
The Future of AI-Powered UX Research Tools
As AI continues to evolve, UX research tools will become even more powerful:
- Real-time analysis: AI will offer more advanced real-time insights, allowing teams to make faster design changes.
- Deeper personalization: AI tools will enable more granular personalization, creating highly tailored user experiences for every individual.
AI-Driven User Experience for Virtual and Augmented Reality (VR/AR)
In the future, AI-powered tools will play a crucial role in UX research for VR and AR environments:
- Optimizing immersive experiences: AI will help design more intuitive and interactive interfaces for VR/AR, improving engagement in these emerging platforms.
Conclusion
AI-powered tools are revolutionizing UX research, offering product managers and designers faster insights, deeper analytics, and more personalized user experiences. By integrating AI into UX workflows, teams can not only optimize designs but also align with broader business goals, such as improving user retention, accelerating product development, and driving revenue growth.
As AI technology continues to evolve, it will become even more integral to delivering user experiences that are not only intuitive and efficient but also aligned with the strategic vision of the business.